mining smart card data for transit riders travel patterns This paper proposes an efficient and effective data-mining procedure that models the travel patterns of transit riders in Beijing, China. Transit riders' trip chains are identified based on the . China Nfc wholesale - Select 2024 high quality Nfc products in best price from certified Chinese .
0 · Understanding commuting patterns using transit smart card data
1 · Travel Pattern Recognition using Smart Card Data in Public Transit
2 · Probabilistic model for destination inference and travel pattern
3 · Mining smart card data for transit riders’ travel patterns
4 · Mining smart card data for transit riders’ travel
5 · Mining smart card data for transit riders' travel patterns
6 · Mining Smart Card Data for Transit Riders’ Travel Patterns
Amiibo data are stored on the physical Amiibo as a .bin file. .Bin file - raw data from physical Amiibo. .NFC file - the file needed to write to an NFC tag/card or send via nfc to your switch, this emulates a physical Amiibo. Note: You won't .
To this end, we propose a network-constrained temporal distance measure for modeling PT rider travel patterns from smart card data; and further introduce a fully autonomous approach to. A methodology for mining smart card data is developed to recognize the travel patterns of transit riders and adopts the density-based spatial clustering of application with .The authors have proposed an efficient data mining approach to process large amounts of smart card transit data and therefore estimate individual transit user's trip chains and group their . To deal with this data issue, this paper proposes a robust and comprehensive data-mining procedure to extract individual transit riders’ travel patterns and regularity from a large dataset with incomplete information. .
Understanding commuting patterns using transit smart card data
Travel Pattern Recognition using Smart Card Data in Public Transit
This paper proposes an efficient and effective data-mining procedure that models the travel patterns of transit riders in Beijing, China. Transit riders' trip chains are identified based on the . This study develops a series of data mining methods to identify the spatiotemporal commuting patterns of Beijing public transit riders. Using one-month transit smart card data, . Therefore, this paper proposes an efficient and effective data-mining approach that models the travel patterns of transit riders using the smart card data collected in Beijing, .
A methodology for mining smart card data is developed to recognize the travel patterns of transit riders and adopts the density-based spatial clustering of application with noise (DBSCAN) .
This paper uses a probabilistic topic model for smart card data destination estimation and travel pattern mining. We establish a three-dimensional LDA model than captures the time, origin, . We proposed an efficient and effective data-mining procedure that models the travel patterns of transit riders using the transit smart card data. Transit riders’ trip chains are identified based on the temporal and spatial characteristics of smart card transaction data.To this end, we propose a network-constrained temporal distance measure for modeling PT rider travel patterns from smart card data; and further introduce a fully autonomous approach to.
how to make rfid blocking credit card sleeves
Probabilistic model for destination inference and travel pattern
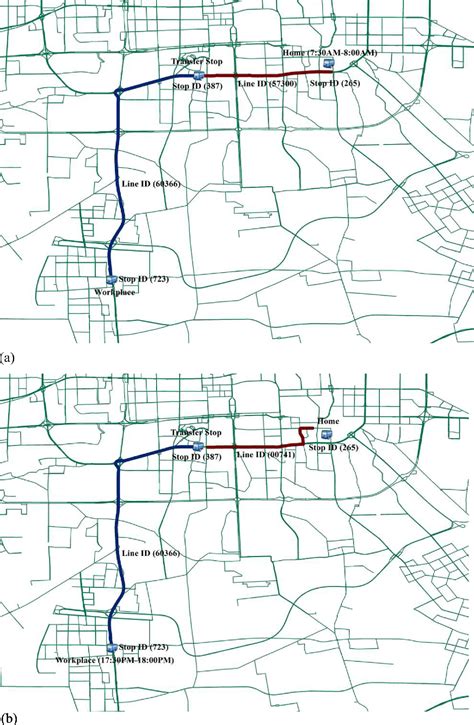
global rfid tags market
A methodology for mining smart card data is developed to recognize the travel patterns of transit riders and adopts the density-based spatial clustering of application with noise (DBSCAN) algorithm to mine the historical travel patterns of each transit riders.The authors have proposed an efficient data mining approach to process large amounts of smart card transit data and therefore estimate individual transit user's trip chains and group their travel pattern regularity.To deal with this data issue, this paper proposes a robust and comprehensive data-mining procedure to extract individual transit riders’ travel patterns and regularity from a large dataset with incomplete information. Specifically, two major issues are examined in this study.This paper proposes an efficient and effective data-mining procedure that models the travel patterns of transit riders in Beijing, China. Transit riders' trip chains are identified based on the temporal and spatial characteristics of their smart card transaction data.
This study develops a series of data mining methods to identify the spatiotemporal commuting patterns of Beijing public transit riders. Using one-month transit smart card data, we measure spatiotemporal regularity of individual commuters, .
Therefore, this paper proposes an efficient and effective data-mining approach that models the travel patterns of transit riders using the smart card data collected in Beijing, China. Transit riders’ trip chains are identified based on the temporal and spatial characteristics of smart card transaction data. Based on the identified trip chains .A methodology for mining smart card data is developed to recognize the travel patterns of transit riders and adopts the density-based spatial clustering of application with noise (DBSCAN) algorithm to mine the historical travel patterns of each transit riders.This paper uses a probabilistic topic model for smart card data destination estimation and travel pattern mining. We establish a three-dimensional LDA model than captures the time, origin, and destination attributes in smart card trips.
We proposed an efficient and effective data-mining procedure that models the travel patterns of transit riders using the transit smart card data. Transit riders’ trip chains are identified based on the temporal and spatial characteristics of smart card transaction data.To this end, we propose a network-constrained temporal distance measure for modeling PT rider travel patterns from smart card data; and further introduce a fully autonomous approach to. A methodology for mining smart card data is developed to recognize the travel patterns of transit riders and adopts the density-based spatial clustering of application with noise (DBSCAN) algorithm to mine the historical travel patterns of each transit riders.
Mining smart card data for transit riders’ travel patterns
The authors have proposed an efficient data mining approach to process large amounts of smart card transit data and therefore estimate individual transit user's trip chains and group their travel pattern regularity.
To deal with this data issue, this paper proposes a robust and comprehensive data-mining procedure to extract individual transit riders’ travel patterns and regularity from a large dataset with incomplete information. Specifically, two major issues are examined in this study.
This paper proposes an efficient and effective data-mining procedure that models the travel patterns of transit riders in Beijing, China. Transit riders' trip chains are identified based on the temporal and spatial characteristics of their smart card transaction data.
This study develops a series of data mining methods to identify the spatiotemporal commuting patterns of Beijing public transit riders. Using one-month transit smart card data, we measure spatiotemporal regularity of individual commuters, . Therefore, this paper proposes an efficient and effective data-mining approach that models the travel patterns of transit riders using the smart card data collected in Beijing, China. Transit riders’ trip chains are identified based on the temporal and spatial characteristics of smart card transaction data. Based on the identified trip chains .A methodology for mining smart card data is developed to recognize the travel patterns of transit riders and adopts the density-based spatial clustering of application with noise (DBSCAN) algorithm to mine the historical travel patterns of each transit riders.
iot rfid tags

Download the Blue Social App from the App Store or Google Play. When opening it for the first .
mining smart card data for transit riders travel patterns|Mining smart card data for transit riders’ travel