mining smart card data for transit riders travel patterns The authors have proposed an efficient data mining approach to process large amounts of smart card transit data and therefore estimate individual transit user's trip chains and group their . iPhone Screenshots. NFC Tap is your all-in-one solution for reading and writing NFC chips, designed with a user-friendly interface for smooth operation. Just bring your device close to .
0 · Understanding commuting patterns using transit smart card data
1 · Travel Pattern Recognition using Smart Card Data in Public Transit
2 · Probabilistic model for destination inference and travel pattern
3 · Mining smart card data for transit riders’ travel patterns
4 · Mining smart card data for transit riders’ travel
5 · Mining smart card data for transit riders' travel patterns
6 · Mining Smart Card Data for Transit Riders’ Travel Patterns
This item: 35 Pcs NFC Mini Cards Compatible with TOTK and BOTW -with Iron .I don't often suggest this, but shoot, if they aren't making enough, go ahead and buy those 3rd party NFC chips people load with the data for and put inside cards so you can buy them cheap and get .
Understanding commuting patterns using transit smart card data
To this end, we propose a network-constrained temporal distance measure for modeling PT rider travel patterns from smart card data; and further introduce a fully autonomous approach to. A methodology for mining smart card data is developed to recognize the travel patterns of transit riders and adopts the density-based spatial clustering of application with .The authors have proposed an efficient data mining approach to process large amounts of smart card transit data and therefore estimate individual transit user's trip chains and group their .
To deal with this data issue, this paper proposes a robust and comprehensive data-mining procedure to extract individual transit riders’ travel patterns and regularity from a large dataset with incomplete information. .
Travel Pattern Recognition using Smart Card Data in Public Transit
Probabilistic model for destination inference and travel pattern
This paper proposes an efficient and effective data-mining procedure that models the travel patterns of transit riders in Beijing, China. Transit riders' trip chains are identified based on the . This study develops a series of data mining methods to identify the spatiotemporal commuting patterns of Beijing public transit riders. Using one-month transit smart card data, . Therefore, this paper proposes an efficient and effective data-mining approach that models the travel patterns of transit riders using the smart card data collected in Beijing, .
A methodology for mining smart card data is developed to recognize the travel patterns of transit riders and adopts the density-based spatial clustering of application with noise (DBSCAN) .
This paper uses a probabilistic topic model for smart card data destination estimation and travel pattern mining. We establish a three-dimensional LDA model than captures the time, origin, . We proposed an efficient and effective data-mining procedure that models the travel patterns of transit riders using the transit smart card data. Transit riders’ trip chains are identified based on the temporal and spatial characteristics of smart card transaction data.To this end, we propose a network-constrained temporal distance measure for modeling PT rider travel patterns from smart card data; and further introduce a fully autonomous approach to.
A methodology for mining smart card data is developed to recognize the travel patterns of transit riders and adopts the density-based spatial clustering of application with noise (DBSCAN) algorithm to mine the historical travel patterns of each transit riders.The authors have proposed an efficient data mining approach to process large amounts of smart card transit data and therefore estimate individual transit user's trip chains and group their travel pattern regularity.
nfc payment debit card
To deal with this data issue, this paper proposes a robust and comprehensive data-mining procedure to extract individual transit riders’ travel patterns and regularity from a large dataset with incomplete information. Specifically, two major issues are examined in this study.
This paper proposes an efficient and effective data-mining procedure that models the travel patterns of transit riders in Beijing, China. Transit riders' trip chains are identified based on the temporal and spatial characteristics of their smart card transaction data. This study develops a series of data mining methods to identify the spatiotemporal commuting patterns of Beijing public transit riders. Using one-month transit smart card data, we measure spatiotemporal regularity of individual commuters, . Therefore, this paper proposes an efficient and effective data-mining approach that models the travel patterns of transit riders using the smart card data collected in Beijing, China. Transit riders’ trip chains are identified based on the temporal and spatial characteristics of smart card transaction data. Based on the identified trip chains .A methodology for mining smart card data is developed to recognize the travel patterns of transit riders and adopts the density-based spatial clustering of application with noise (DBSCAN) algorithm to mine the historical travel patterns of each transit riders.
This paper uses a probabilistic topic model for smart card data destination estimation and travel pattern mining. We establish a three-dimensional LDA model than captures the time, origin, and destination attributes in smart card trips. We proposed an efficient and effective data-mining procedure that models the travel patterns of transit riders using the transit smart card data. Transit riders’ trip chains are identified based on the temporal and spatial characteristics of smart card transaction data.
To this end, we propose a network-constrained temporal distance measure for modeling PT rider travel patterns from smart card data; and further introduce a fully autonomous approach to. A methodology for mining smart card data is developed to recognize the travel patterns of transit riders and adopts the density-based spatial clustering of application with noise (DBSCAN) algorithm to mine the historical travel patterns of each transit riders.The authors have proposed an efficient data mining approach to process large amounts of smart card transit data and therefore estimate individual transit user's trip chains and group their travel pattern regularity.
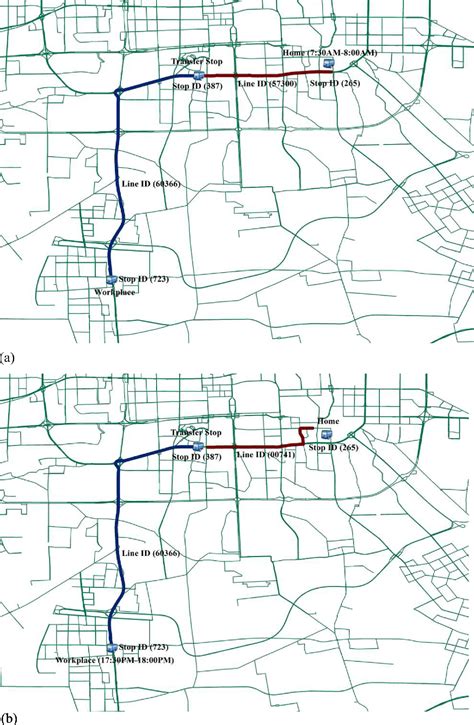
To deal with this data issue, this paper proposes a robust and comprehensive data-mining procedure to extract individual transit riders’ travel patterns and regularity from a large dataset with incomplete information. Specifically, two major issues are examined in this study.
This paper proposes an efficient and effective data-mining procedure that models the travel patterns of transit riders in Beijing, China. Transit riders' trip chains are identified based on the temporal and spatial characteristics of their smart card transaction data.
This study develops a series of data mining methods to identify the spatiotemporal commuting patterns of Beijing public transit riders. Using one-month transit smart card data, we measure spatiotemporal regularity of individual commuters, . Therefore, this paper proposes an efficient and effective data-mining approach that models the travel patterns of transit riders using the smart card data collected in Beijing, China. Transit riders’ trip chains are identified based on the temporal and spatial characteristics of smart card transaction data. Based on the identified trip chains .
A methodology for mining smart card data is developed to recognize the travel patterns of transit riders and adopts the density-based spatial clustering of application with noise (DBSCAN) algorithm to mine the historical travel patterns of each transit riders.
Mining smart card data for transit riders’ travel patterns
Explore NFC tutorial covering NFC protocol stack, how NFC works, frame structure and Near Field Communication modes used for seamless wireless communication.
mining smart card data for transit riders travel patterns|Understanding commuting patterns using transit smart card data